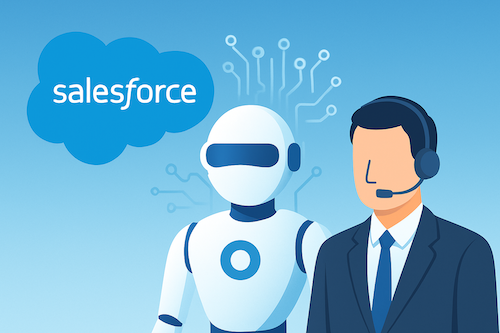
Table of Contents
The conversation surrounding artificial intelligence within enterprise settings is rapidly evolving, moving beyond basic automation towards genuinely autonomous systems. While chatbots have certainly become a familiar sight, the advent of sophisticated AI agents signals a profound maturation in this technology. Salesforce, with its Agentforce platform, is clearly aiming to lead this charge, offering capabilities that promise to extend far beyond simple query resolution. A perspective forged through years of navigating real-world enterprise integrations suggests these agents could fundamentally alter how businesses conceptualize automation, customer engagement, and internal operational flows.
Beyond Chatbots: What Are AI Agents?
It’s important to distinguish these new AI agents from their simpler chatbot predecessors. Traditional chatbots often operate on predefined scripts and decision trees. In contrast, platforms like Agentforce enable agents designed for autonomy. These systems leverage machine learning and natural language processing to perceive their environment (primarily through data), reason through complex requests, plan multi-step actions, and execute tasks. Crucially, they are designed to learn and adapt from interactions over time.
Think of them less like interactive FAQs and more like specialized digital co-workers capable of handling complex workflows. Their power stems from deep integration with underlying data platforms, such as Salesforce’s Data Cloud, allowing them to access and act upon a unified view of customer and operational information in real time. This level of integration is something I’ve seen as a critical success factor in past technological shifts.
Potential Capabilities Across the Enterprise
The advertised use cases for Agentforce are broad, touching nearly every facet of a CRM-driven business. From an analytical perspective, drawing upon insights distilled from numerous complex system deployments, several areas stand out with significant potential:
- Advanced Service Automation: Agents are shown handling tasks like order modifications, return processing, complex billing inquiries, and technical troubleshooting. This suggests a move beyond deflecting simple queries towards resolving substantive issues, potentially improving service efficiency and consistency.
- Sales and Revenue Operations: Use cases include lead qualification, meeting scheduling, answering complex product questions, and even providing predictive analytics on sales trends or client health scores. The Cloud23 example, building an AI agent to perform many functions of a Chief Revenue Officer, illustrates the ambition – automating analysis and strategic tasks, not just routine communication.
- Operational & Data Management: Agents are also described performing internal tasks, including data processing, synchronization between systems, and managing data discrepancies. This points towards their potential role in enhancing data quality and streamlining back-office functions often associated with enterprise systems.
While this capability spectrum is impressive, it’s a significant leap from the task-specific automation offered by technologies like Robotic Process Automation (RPA). Longitudinal data and field-tested perspectives highlight that such leaps often come with underappreciated adoption hurdles.
Navigating the Hype Cycle: Lessons from Past Transformations
It’s not uncommon to see cutting-edge technologies like Agentforce generate considerable excitement. My years observing enterprise technology adoption cycles, from the initial ERP booms to the more recent cloud migrations, have shown a recurring pattern: initial enthusiasm often outpaces the practical realities of implementation. For instance, I recall numerous situations where the promise of a unified platform met the stubborn complexities of siloed departmental processes and legacy data structures. These weren’t failures of the technology itself, but rather a reflection of the immense organizational effort required to prepare for and leverage such transformative tools.
The journey with AI agents will likely echo these past experiences. While the potential for these “digital co-workers” is undeniable, organizations would do well to temper expectations with a healthy dose of realism regarding the foundational work needed. This isn’t to diminish the potential, but to frame it within a pragmatic understanding of enterprise change.
The Criticality of Foundations: Data and Process
However, the potential of these agents hinges entirely on the foundation upon which they are built. Research and best practices highlighted in documentation (like that from Elements.cloud regarding Agentforce implementation) consistently emphasize two prerequisites:
- High-Quality, Governed Data: An agent acting autonomously based on flawed, incomplete, or inconsistent data is a recipe for errors and poor outcomes. Robust data governance frameworks and clean, unified data (the promise of Data Cloud) are non-negotiable. The Einstein Trust Layer, with features for PII masking and ethical use, is presented as a necessary safeguard.
- Well-Defined Processes: Agents need clear instructions and workflows. Simply unleashing an AI on poorly understood or undocumented processes is unlikely to yield positive results. Methodologies like Universal Process Notation (UPN) are cited as valuable for mapping workflows before attempting automation with agents.
Strategic Implications for Businesses
The rise of capable AI agents, such as those heralded by Agentforce, presents several strategic considerations for businesses. The allure of substantial efficiency gains and cost reductions (as hinted at by initiatives like the Cloud23 ROI claims) is undeniably strong. Yet, true success in adoption will extend beyond mere software procurement. It necessitates a deeply strategic commitment to data management, meticulous process definition, and robust governance. Organizations must undertake candid assessments of their current readiness, articulate clear, achievable objectives, and strategically initiate with manageable, high-impact use cases.
This technology represents another major step in digital transformation, pushing the boundaries of automation further into the realm of complex reasoning and task execution within core enterprise systems. It’s a development that many of us in the field have anticipated, watching the steady convergence of AI capabilities with enterprise needs.
Charting the Course Forward
Salesforce Agentforce indeed signals a future where AI is poised to assume a more autonomous and integral role within the fabric of business operations. The potential benefits are clearly substantial. However, unlocking them will invariably demand meticulous planning, an unshakeably solid data foundation, and rigorously defined processes. This is an evolutionary domain that warrants close, ongoing observation as organizations begin to navigate this next significant wave of enterprise AI.
What are your thoughts on the potential impact of autonomous AI agents in the enterprise? Join the discussion on LinkedIn.