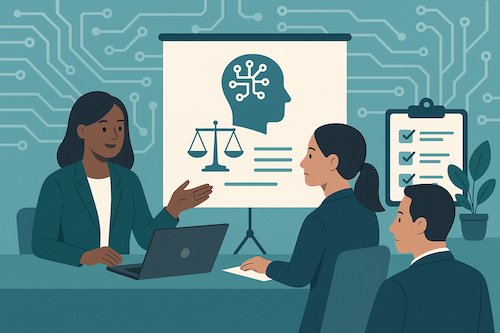
Table of Contents
The relentless march of Artificial Intelligence into the core of enterprise systems isn’t just a trend; it’s a fundamental reshaping of how businesses operate. As we look towards 2025, the conversation must pivot from mere adoption to deep-seated ethical integration. Why? Because without a sturdy governance framework, the very tools designed for progress can introduce unforeseen risks. This isn’t merely about ticking compliance boxes; it’s about embedding trust and ensuring that technological advancements align with enduring human values. A perspective forged through years of navigating real-world enterprise integrations suggests this foresight is no longer optional, but essential.
The Specter of Data Bias: Unearthing and Mitigating Hidden Risks
At the heart of many AI systems lies data, vast oceans of it. Yet, what if this foundational data reflects historical prejudices or skews? The AI, in its digital diligence, will learn and perpetuate these biases. We’ve seen this play out: hiring algorithms that inadvertently favor one demographic, or customer service bots that are less effective for certain user groups. Insights distilled from numerous complex system deployments indicate that these biases are often subtle, woven into the fabric of legacy data.
Mitigation isn’t a simple affair. It demands a proactive strategy:
- Rigorous Data Audits: Scrutinizing training datasets for imbalances and ingrained prejudices.
- Diverse Data Sourcing: Actively seeking out and incorporating data that represents a broader spectrum of experiences and populations.
Continuous monitoring of AI outputs for discriminatory patterns is also paramount. This isn’t a one-and-done task but an ongoing commitment to fairness.
Illuminating the Black Box: Fostering Transparency in AI Decisions
“The system decided” is no longer an acceptable explanation, especially when decisions significantly impact individuals or business outcomes. Many AI models, particularly deep learning networks, can operate as “black boxes,” their internal workings opaque even to their creators. How do we build trust if we can’t understand the rationale?
The push for eXplainable AI (XAI) is a direct response. For enterprise applications, transparency involves developing systems capable of articulating the “why” behind their recommendations or actions. This could mean providing simplified logic trails, highlighting key influencing factors, or offering confidence scores. Clear documentation of model design, data lineage, and the parameters influencing decisions is fundamental. Stakeholders, and sometimes even customers, need avenues to understand and, if necessary, challenge AI-driven conclusions. This is where complex technology must gracefully interface with human oversight.
Accountability in an Automated World: Defining Responsibility
When an AI system falters, perhaps a predictive maintenance model fails, leading to costly downtime, or an automated financial advisory tool gives poor counsel, who bears responsibility? Is it the algorithm’s developers, the providers of the data it trained on, or the organization that deployed it? Without clear accountability structures, we risk a scenario where responsibility diffuses into ambiguity.
A robust governance framework must clearly delineate roles. This includes establishing an AI ethics board or review panel, defining processes for redress when AI makes an error, and strategically embedding human-in-the-loop (HITL) interventions for critical decision junctures. Longitudinal data and field-tested perspectives highlight the crucial nature of these structures to prevent systemic drift and ensure that automated systems remain answerable. It’s less about assigning blame and more about building resilient systems that learn from missteps.
Navigating the Shifting Sands: The Evolving Regulatory Landscape
The regulatory environment surrounding AI is, to put it mildly, dynamic. Jurisdictions globally are grappling with how to foster innovation while safeguarding against AI’s potential harms. While specifics vary, common themes are emerging: demands for fairness, transparency, security, data privacy, and accountability.
Waiting for definitive legislation before acting is a risky game. Organizations should instead aim to build agile governance frameworks that can adapt to new legal requirements. This involves embedding core ethical principles into AI development and deployment lifecycles now. Think of it as future-proofing your AI strategy. It’s a bit of a tightrope walk, balancing proactive compliance with the drive for innovation, isn’t it?
Weaving Ethics into the Enterprise DNA
Ultimately, ethical AI isn’t a specialized department or a supplementary checklist. It must become an intrinsic part of an organization’s operational fabric and strategic vision. This cultural shift requires commitment from leadership, ongoing education for employees, and fostering collaboration across typically siloed units (such as IT, legal, HR, and core business functions).
The objective is clear: to harness the transformative power of AI in a manner that is not only effective but also responsible, equitable, and aligned with the organization’s core values. The journey to ethical AI is continuous, demanding vigilance and a readiness to adapt.
Want to discuss these challenges further or share your organization’s approach? I welcome you to connect with me on LinkedIn.