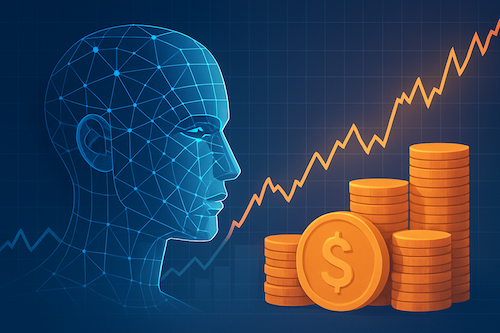
Table of Contents
Financial technology’s churn is relentless, isn’t it? Yet, generative AI feels like a different beast altogether compared to past innovations. We’re talking about a technology poised to reshape financial markets in ways we’re only starting to grasp, with serious implications for trading, asset management, and the bedrock of systemic stability.
The Bank of England’s recent Financial Stability in Focus report (April 2025) throws a necessary spotlight on this. It digs into how AI isn’t just boosting productivity but is actively transforming financial decision-making, often introducing sneaky new systemic vulnerabilities. It’s not just about faster number crunching; it’s about fundamental shifts in how capital navigates our financial ecosystem.
AI Models: Turbocharging Trading, But At What Cost?
Think about trading floors (or, more accurately these days, server rooms). Books like “Generative AI for Trading and Asset Management” by Medina and Chan really dive into how AI transforms trading via sophisticated pattern recognition and even generating novel strategies. What jumped out during my research, though, was the double-edged nature of this power. Sure, AI can sniff out inefficiencies and potentially tighten spreads, making markets seem smoother. But what happens when too many players use similar AI models trained on overlapping data?
This feeds directly into the Bank of England’s worry about “correlated positioning.” It’s a fancy term for a simple, dangerous idea: AI-driven strategies, often opaque even to their users, might inadvertently push herds of investors in the same direction, especially during stress events. The report warns that “firms taking increasingly correlated positions and acting in a similar way during a stress” could blow up financial stability risks. It’s the kind of risk individual firms, optimizing their own little corners, might totally miss in their models until it’s too late. Remember Long-Term Capital Management? That was human groupthink; AI groupthink could move far faster.
The Core of Decision Making: AI in the Driver’s Seat?
Now, let’s shift to core banking. When AI gets embedded into credit decisions or risk assessments, we hit another potential snag. Model risk isn’t exactly new territory – banks have wrestled with validating traditional statistical models for ages. But the dynamic, often self-learning nature of advanced AI models? That presents unique validation headaches. The Financial Policy Committee (FPC) nails it when they identify that “common weaknesses in widely used models cause many firms to misestimate certain risks.” It’s a recipe for potentially systemic mispricing of assets or risks.
The sheer technical complexity here makes me wonder about the regulatory lag. How can supervisors effectively challenge a model that even its creators might not fully understand? When multiple major institutions start relying on similar underlying datasets or off-the-shelf model architectures (because building these things from scratch is hard), we’re not distributing risk anymore. We’re concentrating it, setting the stage for synchronized failures instead of isolated ones. It’s a different kind of domino effect.
The Concentration Conundrum: Betting the Farm on a Few AI Giants
Perhaps the most structurally concerning trend is the operational dependency forming around a handful of large AI service providers. The Bank of England flags this clearly: “reliance on a small number of providers for a given service could lead to systemic risks.” This isn’t just about software bugs; it’s about market power and single points of failure.
Think about it: training cutting-edge large language models or sophisticated trading AIs requires massive computational resources and specialized expertise. This naturally favors a few dominant tech players (you can probably guess who). Financial institutions, eager for an edge or just to keep up, might increasingly rely on these external providers for critical functions. What happens if one of these providers suffers a major outage, a security breach, or even subtly changes its algorithms? The ripple effects across the financial system could be immense. Traditional financial risk frameworks just weren’t built for this kind of concentrated, tech-layer dependency.
Charting the Course: Adaptability is Key
So, what’s the path forward? Panicking won’t help. Instead, we need relentless monitoring and adaptation. The Bank of England’s plan to use various information channels – surveys, supervisory intelligence, market contacts – seems like a sensible starting point. It’s about keeping eyes and ears open. But I still question whether traditional regulatory toolkits, often designed for slower-moving risks, can truly keep pace with AI’s breakneck evolution. Can yearly stress tests capture risks that evolve weekly?
Understanding these emerging systemic knots – the hidden correlations, the model opacity, the provider concentration – needs to be front-and-center in any firm’s AI adoption strategy. It’s not just about chasing efficiency gains. Risk frameworks need a serious upgrade to account for these new interconnections.
The siren song of AI-driven productivity is powerful, no doubt. But navigating this transition demands a clear-eyed view of the intricate, and sometimes fragile, new plumbing being installed in our global financial system. We need to embrace the potential while actively managing the novel risks it introduces.
What are your thoughts on the intersection of generative AI and financial stability? Let's connect on LinkedIn to discuss further.